-
Governance advisory
We guide boards and management teams in frameworks, team processes and leadership dynamics to deliver sustainable value.
-
Financial services advisory
Get market-driven expertise to achieve your goals in banking, insurance, capital markets, and investment management.
-
Business risk services
Our market-driven expertise helps firms keep growing and manage risk in an evolving regulatory landscape.
-
Risk
Meet risks with confidence and transform your business – we support you to manage risk and deliver on your goals.
-
Economic consulting
Bespoke guidance grounded in complex economic theory and practical sector insight to help you make the right decisions.
-
Government and public sector
Experience and expertise in delivering quality public sector advisory and audits.
-
Business consulting
Partnering with you to deliver sustainable business change that helps you realise your ambitions.
-
Transaction advisory services
Whether buying or selling, we help you get the deal done with our comprehensive range of transaction advisory services.
-
CFO solutions
Our CFO solutions team can support your finance function with the flexible resource they need to get results.
-
Corporate finance advisory
Building a business is never easy. We help you maximise the value of your business and find the right option.
-
Valuations
Help to understand or support the valuation of a business or asset.
-
Insolvency and global asset recovery
We provide asset tracing and seamless cross-border global recovery for clients.
-
Forensic and investigation services
Market-driven expertise in investigations, dispute resolution and digital forensics.
-
Restructuring
Our restructuring team help lenders, investors and management navigate contingency plans, restructuring and insolvency.
-
Transformation consulting
Is business transformation a priority for your organisation? Our expert insight and guidance can help you achieve it.
-
Pensions assurance
A tailored service that responds to evolving risks and regulations.
-
Accounting services
Optimise your growth with expert accounting services. Contact us today.
-
Royalty and intellectual property (IP) audits
Enhance IP asset protection with our royalty and IP audit services. Expertise in licensing, revenue detection, and compliance improvements.
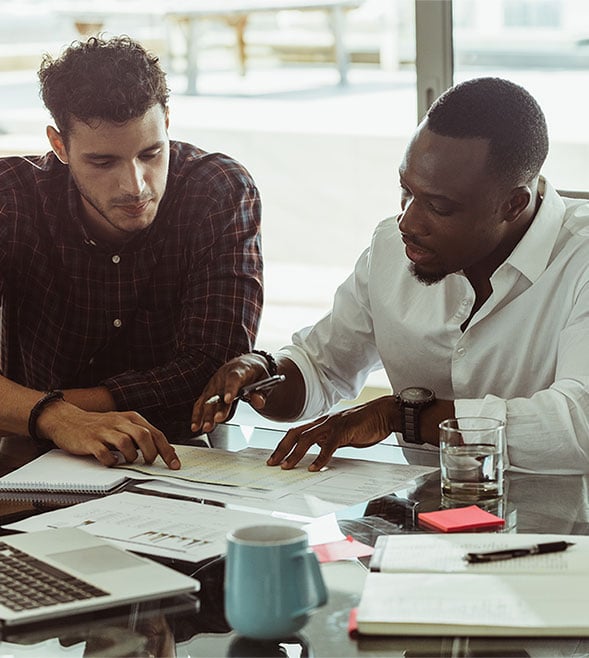
-
Business consulting
Partnering with you to deliver sustainable business change that helps you realise your ambitions.
-
CFO solutions
Our CFO solutions team can support your finance function with the flexible resource they need to get results.
-
Corporate Simplification
Release value, reduce compliance complexity, and improve tax efficiency by streamlining your group structure.
-
Economic consulting
Bespoke guidance grounded in complex economic theory and practical sector insight to help you make the right decisions.
-
Governance advisory
We guide boards and management teams in frameworks, team processes and leadership dynamics to deliver sustainable value.
-
International
Unlock global opportunities with our local expertise and worldwide reach.
-
People advisory
Driving business performance through people strategy and culture.
-
Strategy Group
Successful business strategy is rooted in a clear understanding of the market, customer segmentation and how purchase decisions vary.
-
Respond: Data breach, incident response and computer forensics
Are you prepared for a cyber failure? We can help you avoid data breaches and offer support if the worst happens.
-
Comply: Cyber security regulation and compliance
Cyber security regulation and compliance is constantly evolving. Our team can support you through the digital landscape.
-
Protect: Cyber security strategy, testing and risk assessment
Cyber security threats are constantly evolving. We’ll work with you to develop and test robust people, process and technology defences to protect your data and information assets.
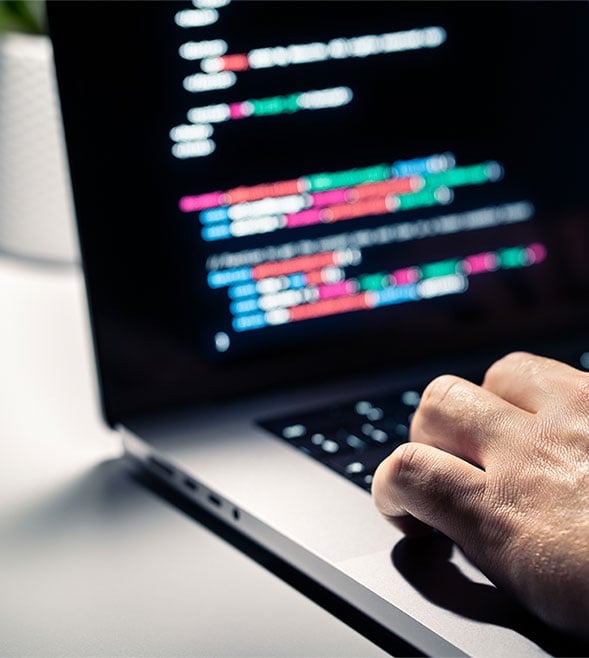
-
Corporate finance advisory
Building a business is never easy. We help you maximise the value of your business and find the right option.
-
Debt advisory
Working with borrowers and private equity financial sponsors on raising and refinancing debt. We can help you find the right lender and type of debt products.
-
Financial modelling services
Financial modelling that helps you wrestle with your most pressing business decisions.
-
M&A data analytics
We transform and visualise data to present meaningful and clear outputs, enabling you to make better decisions and realise greater value.
-
Operational deal services
Enabling transaction goals through due diligence, integration, separation, and other complex change.
-
Our credentials
Search our transactions to see our experience in your sector and explore the deals advisory services we've delivered.
-
Transaction advisory services
Whether buying or selling, we help you get the deal done with our comprehensive range of transaction advisory services.
-
Valuations
Help to understand or support the valuation of a business or asset.
-
The ESG agenda
Shape your ESG agenda by identifying the right metrics, sustainable development and potential business value impact.
-
ESG driven business transition
Whatever your ESG strategy, we can support your organisation as it evolves while maximising efficiency and profitability.
-
ESG programme and change management
Do you have the right capabilities to drive the delivery of your ESG strategy to realise your targets?
-
ESG risk management
You must protect, comply, understand and influence to successfully manage the risk involved with ESG issues. We can help.
-
ESG strategy, risk and opportunity identification
We can help you clearly define your ESG Strategy, with the risks and opportunities identified and managed.
-
Create value through effective ESG communication
Building trust and engagement with your stakeholders on your ESG strategy.
-
ESG metrics, targets and disclosures
The pressure to report your ESG progress is growing. Do your targets measure up?
-
ESG governance, leadership and culture framework
Make the most of ESG opportunities by effectively embedding your strategy across your organisation.
-
ESG and non-financial assurance
Support your board to be confident in supplying robust information that withstands scrutiny.
-
Transition planning to net zero
Supporting your organisation in the transition to net zero.
-
Actuarial and insurance consulting
We consult extensively to the life insurance, general insurance, health insurance and pensions sectors.
-
Business risk services
Our market-driven expertise helps firms keep growing and manage risk in an evolving regulatory landscape.
-
Financial crime
Helping you fight financial crime in a constantly changing environment
-
Financial services business consulting
Leverage our diverse capabilities to manage challenges and take opportunities: from assurance to transformation
-
Financial services tax
Helping financial services firms navigate the global financial services and funds tax landscape.
-
Regulatory and compliance
Providing an exceptional level of regulatory and compliance to firms across the financial services industry.
-
Corporate intelligence
Corporate intelligence often involves cross-border complexities. Our experienced team can offer support.
-
Litigation support
Industry-wide litigation support and investigation services for lawyers and law firms.
-
Disputes advisory
Advising on quantum, accounting and financial issues in commercial disputes.
-
Forensic investigations and special situations
Do you need clarity in an uncertain situation? If you're accused of wrongdoing we can help you get the facts right.
-
Forensic data analytics
Our forensic data analytics team are helping businesses sift the truth from their data. See how we can help your firm.
-
Monitoring trustee and competition services
Monitoring trustee services to competition, financial and regulatory bodies.
-
Financial crime
Supporting your fight against financial crime in an ever-changing environment
-
Whistleblowing and investigation support
Whistleblowing frameworks provide many benefits – find out how to build trust and manage risks in a confidential, cost-effective, robust way.
-
Public sector advisory
To deliver excellent public services, local and central government need specialist support.
-
Public sector consulting
Helping public sector organisations maintain oversight of services and understand what's happening on the ground.
-
Public sector audit and assurance
As a leading UK auditor, we have unparalleled insights into the risks, challenges and opportunities that you face.
-
Competition damages and class actions
Helping you recover damages owed from anti-competitive practices.
-
Contentious estates and family disputes
We manage complex and sensitive disputes through to resolution.
-
Digital Asset Recovery
Get guidance and technical expertise on digital finance and cryptoasset recovery from our dedicated crypto hub.
-
Grant Thornton Offshore
Grant Thornton Offshore is our one-stop global solution for insolvency, asset recovery, restructuring and forensics services.
-
Insolvency Act Portal
Case information and published reports on insolvency cases handled by Grant Thornton UK LLP.
-
Litigation support
Industry-wide litigation support and investigation services for lawyers and law firms.
-
Personal insolvency
We can support you to maximise personal insolvency recovery and seek appropriate debt relief.
-
South Asia business group
We help Indian companies expand into the UK and invest globally. We also help UK companies invest and operate in India.
-
US business group
Optimise your trans-Atlantic operations with local knowledge and global reach.
-
Japan business group
Bridging the commercial and cultural divide and supporting your ambitions across Japan and the UK.
-
Africa business group
Connecting you to the right local teams in the UK, Africa, and the relevant offshore centres.
-
China-Britain business group
Supporting your operations across the China – UK economic corridor.
-
Asset based lending advisory
Helping lenders, their clients and other stakeholders navigate the complexities of ABL.
-
Contingency planning, administrations and liquidations
In times of financial difficulty, it is vital that directors explore all the options that are available to them, including having a robust ‘Plan B’.
-
Corporate restructuring
Corporate restructuring can be a difficult time. Let our team make the process simple and as stress-free as possible.
-
Creditor and lender advisory
Whether you're a creditor or lender, complex restructurings depend on pragmatic commercial advice
-
Debt advisory
Our debt advisory team can find the right lender to help you in restructuring. Find out how our experts can support you.
-
Financial services restructuring and insolvency
Financial services restructuring and insolvency is a competitive marketplace. Our team can help you navigate this space.
-
Pensions advisory services
DB pension-schemes need a balanced approach that manages risk for trustees and sponsors in an uncertain economy.
-
Restructuring and insolvency tax
Tax will often be crucial in a plan to restructure a distressed business. Our team can guide you through the process.
-
Restructuring Plans
Market leading experience in advising companies and creditors in Restructuring Plan processes.
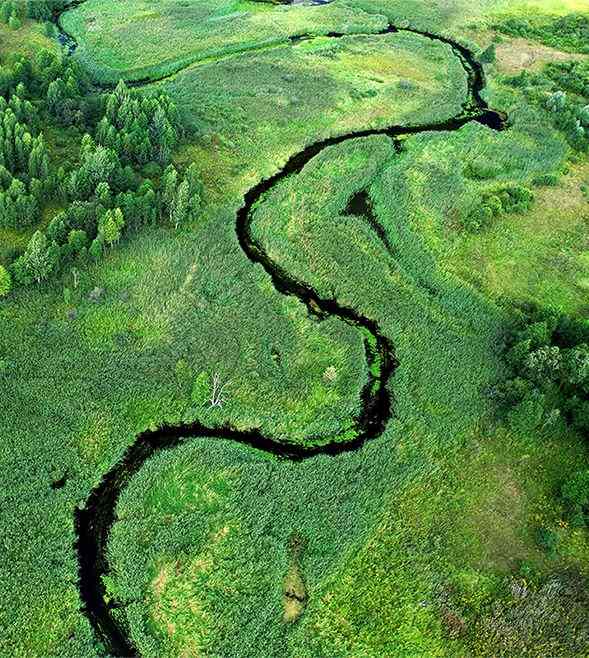
-
Artificial intelligence
Our approach to the design, development, and deployment of AI systems can assist with your compliance and regulation.
-
Controls advisory
Build a robust internal control environment in a changing world.
-
Data assurance and analytics
Enhancing your data processes, tools and internal capabilities to help you make decisions on managing risk and controls.
-
Enterprise risk management
Understand and embrace enterprise risk management – we help you develop and connect risk thinking to your objectives.
-
Internal audit services
Internal audit services that deliver the value and impact they should.
-
Managing risk and realising ESG opportunities
Assess and assure risk and opportunities across ESG with an expert, commercial and pragmatic approach.
-
Project, programme, and portfolio assurance
Successfully delivering projects and programmes include preparing for the wider impact on your business.
-
Service organisation controls report
Independent assurance provides confidence to your customers in relation to your services and control environment.
-
Supplier and contract assurance
Clarity around key supplier relationships: focusing on risk, cost, and operational performance.
-
Technology risk services
IT internal audits and technology risk assurance projects that help you manage your technology risks effectively.
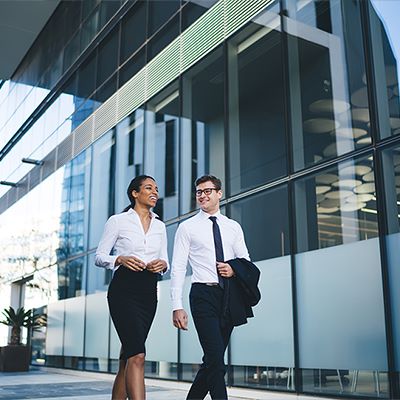
-
Capital allowances (tax depreciation)
Advisory and tools to help you realise opportunities in capital allowances.
-
Corporate tax
Helping companies manage corporate tax affairs: delivering actionable guidance to take opportunities and mitigate risk.
-
Employer solutions
We will help you deliver value through your employees, offering pragmatic employer solutions to increasing costs.
-
Financial services tax
Helping financial services firms navigate the global financial services and funds tax landscape.
-
Indirect tax
Businesses face complex ever changing VAT regimes, guidance and legislation. We can help you navigate these challenges.
-
International tax
Real-world international tax advice to help you navigate a changing global tax landscape.
-
Our approach to tax
We advise clients on tax law in the UK and, where relevant, other jurisdictions.
-
Private tax
Tax experts for entrepreneurs, families and private business. For now and the long term.
-
Real estate tax
Stay ahead of real estate tax changes with holistic, tax-efficient solutions.
-
Research and development tax incentives
We can help you prepare optimised and robust research and development tax claims.
-
Tax dispute resolution
We make it simple to stay compliant and avoid HMRC tax disputes
-
Tax risk management
We work with you to develop effective tax risk management strategies.
-
Digital assets
As frontrunners in digital asset advisory, we’re here to help you navigate both the opportunities and challenges in this unique asset class.
-
Skills and training
Get the right support to deliver corporate and vocational training that leads the way in an expanding market.
-
Private education
Insight and guidance for all businesses in the private education sector: from early years to higher education and edtech.
-
Facilities management and property services
Get insight and strategic support to take opportunities that protect resilience and drive UK and international growth.
-
Recruitment
Helping recruitment companies take opportunities to achieve their goals in a market where talent and skills are key.
-
Food and beverage (F&B)
We can help you find the right ingredients for growth in your food and beverage business.
-
Travel, tourism and leisure
Tap into our range of support for travel, tourism and leisure businesses in this period of challenge and change.
-
Retail, e-commerce and consumer products
With multiple challenges and opportunities in the fast-evolving retail sector, make sure you are ready for them.
-
Banking
Our expertise and insight can help you respond positively to long term and emerging issues in the banking sector.
-
Capital markets
2020 is a demanding year for capital markets. Working with you, we're architecting the future of the sector.
-
Insurance
Our experienced expert team brings you technical expertise and insight to guide you through insurance sector challenges.
-
Investment management
Embracing innovation and shaping business models for long-term success.
-
Pensions
Pension provision is an essential issue for employers, and the role of the trustee is becoming increasingly challenging.
-
Payments advisory and assurance
Payment service providers need to respond to rapidly evolving technical innovations and increased regulatory scrutiny.
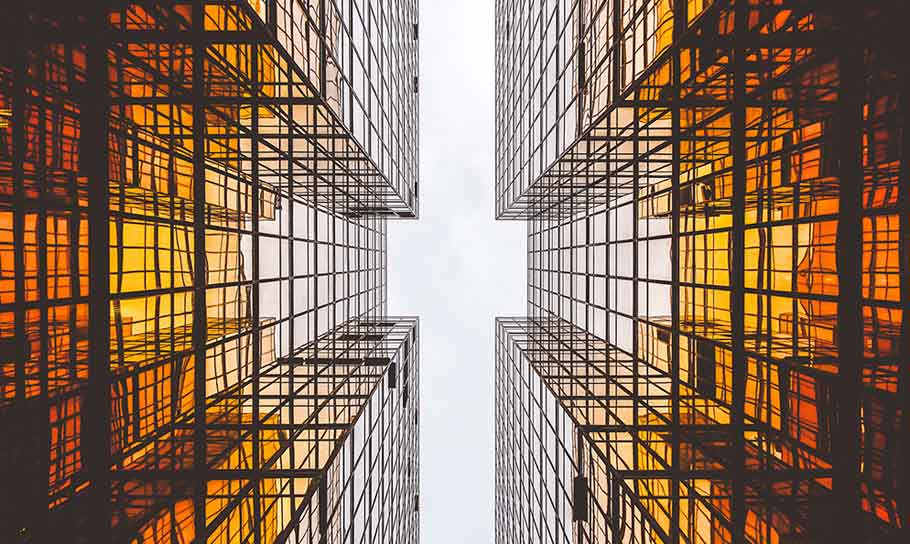
-
Central and devolved government
Helping central and devolved governments deliver change to improve our communities and grow our economies.
-
Infrastructure and transport
Delivering a successful transport or infrastructure project will require you to balance an often complex set of strategic issues.
-
Local government
Helping local government leverage technical and strategic expertise deliver their agendas and improve public services.
-
Regeneration development and housing
We provide commercial and strategic advice to assist your decision making in pursuing your objectives.
-
Health and social care
Sharing insight and knowledge to deliver transformation and improvement to health and social care services.
-
Charities
Supporting you to achieve positive change in the UK charity sector.
-
Education and skills
The education sector has rarely faced more risk or more opportunity to transform. You need to plan for the future.
-
Social housing
We are committed to helping change social housing for the better, and can help you make the most of every opportunity.
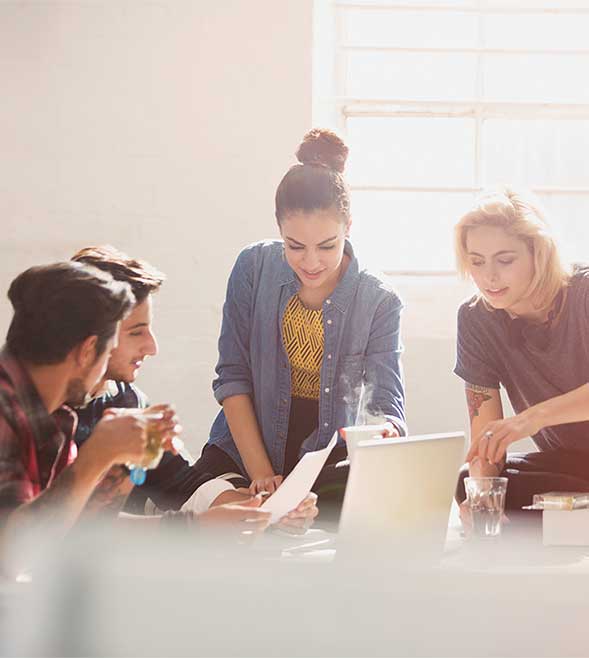
-
Technology
We work with dynamic technology companies of all sizes to help them succeed and grow internationally.
-
Telecommunications
Take all opportunities to realise your goals in telecommunications: from business refresh to international expansion.
-
Media
Media companies must stay agile to thrive in today’s highly competitive market – we’re here to support your ambitions.
-
Career development
The support you need will vary throughout your career, here are just some of the ways we'll support you to you excel with us.
-
How we work
Our approach to how we work, ensures that when we are making choices about how, where or when we work, we have the support and tools we need.
-
Reward and benefits
We are committed to building a culture where our people have access to the necessary benefits to help promote a healthy lifestyle and thrive.
-
Inclusion and diversity
Included and valued for your difference is how everyone should feel at work. Not just because it’s right, but because we’re all at our best when we’re able to be ourselves.
-
ESG: environment and community impact
Our ESG framework enables responsible, sustainable, and ethical operations. We prioritise the environment, our broader societal impact, and our firm's governance to protect the planet, foster inclusivity and wellbeing, support our communities, and bolster our firm's resilience.
-
What we do
It’s an exciting time to be joining Grant Thornton, especially as a trainee at the start of your career. Learn about our teams and the work we do.
-
Life as a trainee
Everything you need to know about life as a trainee, from the experiences you'll get to the skills you need.
-
Employability hub
Our employability hub is designed to help you feel prepared for the application process, and guide you through the decisions you will need to make throughout.
-
Our programmes
Whether you are looking to join us straight from school or with a degree, or even looking for some work experience, we have a programme that is right for you.
-
Parents, carers and teachers hub
We've created this hub to provide you with the information you'll need to help inform career discussions with your young people. From whether an apprenticeship is the right route and the application process, to the support they'll receive and future career opportunities, this page will help you guide informed decisions.
-
FAQs
We hope you can find all the information you need on our website, but to help we've collated a few of the questions we hear quite frequently when speaking to candidates.
-
Advisory
Our advisory practice provides organisations with the advice and solutions they need to unlock sustainable growth and navigate complex risks and challenges.
-
Audit
Every day our audit teams help people in businesses and communities to do what is right and achieve their goals.
-
Tax
The tax landscape is evolving, and our clients need us more than ever to navigate the complexities with them. Our firm has seen remarkable growth in recent years and we have set ambitious plans for the future. To achieve them, we're dedicated to developing our internal talent and excited to welcome new team members who want to grow with us.
-
Central Client Service
From finance and information systems, to marketing and people teams we have a diverse range of teams supporting our business.
-
Our recruitment process
We strive to ensure our interview process is barrier free and sets you up for success, as well as being wholly inclusive and robust. Learn about our process here.
-
International hiring
Our international talent is an important part of our firm, joining us you will be joining a growing community of international hires.
-
Armed forces
As an Armed Forces Friendly organisation we are proud to support members of the Armed Forces Community as they develop their career outside of the Armed Forces.
Regulators are increasingly focused on how firms oversee, govern, and mitigate their model risk. This is reflected in last year’s consultation paper from the Prudential Regulation Authority (PRA), addressing ‘model risk management principles for banks’. Despite the banking focus, the key messaging is equally applicable to insurers and can help you achieve good practice and reduce model risk.
Poor oversight can lead to inaccurate models and limited management information, negatively impacting decision-making processes. There’s also greater potential for financial losses and misstatements, as well as inaccurate regulatory reporting. This can lead to regulatory fines or intervention to remediate issues.
Baselining
There are many ways to manage model risk, and insurers should use methods that work for them. However, you should always consider if your approaches are fit for purpose and remain relevant. This is especially important as models and business changes over time. This year in particular, insurers are transitioning to IFRS 17, and some will have developed models in haste. Insurers will want to guarantee that model risk is managed around IFRS 17 as the dust from implementation settles.
Traditionally, model risk management in the first line of defence is through baselining, but there's also increasing focus in the second line in building model validation capabilities. The scope of baselining can vary from insurer to insurer, but there's a tendency to focus on the model logic with the data being assumed to be correct. When baselining a model, it’s important to look beyond the model logic itself to include data, data transformations, and underlying assumptions. While companies may have controls over each component separately, a joined-up view reduces risk of unintended interactions between data and the model.
Before starting a baselining exercise, a review should be performed on any existing documentation on data, transformations, model engine, and past baselining results. This review will inform you of any gaps or areas where more attention should be given during the baselining exercise.
The starting point for a baselining exercise is a dump of all data from the policy administration system. From there, you can take a sample of policies with a range of different features.
What can you do after selecting a sample?
- Reconcile extract data back to policy administration data (in some cases you may need to go to the original policy documents)
- Apply your data transformations to the sample, in line with your documented processes, and compare your findings against actual model point output
- Run the sample through the model and assess the outputs, eg, against a benchmark model or golden source
A typical baseline exercise, based on a best estimate liability (BEL) model, might look like this:
When documenting results from the baseline exercise, you should ensure that expert judgements and conclusions are well documented. Any issues identified should assessed for materiality and an action plan put in place to address them.
What to consider before performing a baseline
Data transformation
Actuarial models rarely use raw data. They rely on transformed data in the form of model point files. Transformations range from simple mappings of gender to a 1 or 0, to complex logic that influence how a policy is modelled. It’s important that model-point transformations are reviewed to make sure they behave as expected. In particular the transformations need to be consistent with how the valuation engine expects the inputs to look. The valuation engine itself ideally shouldn’t contain any data transformations, but sometimes it can happen, particularly in in-house models.
Legacy products
Many insurers may have old product lines, which tend to have poor-quality data and documentation, with multiple inaccuracies and gaps. Portfolio transfers or M&A can be particularly complex if you haven’t simplified it or aligned it with your own data structures. Problems tend to be greater if old product lines are still stored on legacy systems.
End-to-end understanding
Baselining examines end-to-end processes, so it’s essential to understand the full environment the model operates in. This covers the whole valuation process and associated controls, including the source policy data as a key input, through to output from the valuation engine. Understanding the entire process also helps to reduce the risk at handover points, where data moves from one system or process to another.
Sampling approach
You probably won’t be able to baseline every policy in your book, so you’ll need to take a sample that reflects the range of products and features your policies collectively offer. Choosing the policies for inclusion is tricky. While you can use a risk-based approach, it’s important to make sure you don’t introduce any bias – for example, you may overlook policies with a low reserve and data issues in favour of high reserve policies. Ideally, you want a sample that demonstrates treatment of all product features over time, initially focusing on the more complex characteristics.
Critical data
As with the sampling size, you won’t be able validate all fields for model inputs. Once again, a risk-based approach will help you identify the critical data fields with the most material impact on the results. This may require expert judgement or quantitative methods, such as sensitivity analysis. Both approaches have their pros and cons. A risk-based approach is quicker, but expert judgement is subjective. On the other hand, quantitative methods offer greater objectivity but can be a lot of work. Ultimately, you may need a blended approach to maximise the benefits from each. Whichever approach you take, you need to document it appropriately, including any assumptions behind the expert judgement.
Documentation
Firms generally don’t spend long on their model documentation, so it tends to be of limited value for model baselining. This is compounded by the complexity of insurance models, so it’s essential to use simple language when documenting model processes. You could consider replica spreadsheets to record code, or other innovative approaches to make model documents easier to read and understand. While it may take longer to effectively document your model processes, it's worth it. Poor documentation can increase the risk of errors and also pose a problem in terms of succession planning. If the original model builder departs the company or team, poor documentation can leave you without anyone that really understands how the model works. Robust model documentation practices and policies will help you manage model risk and add value to your business.
Resourcing
Baselining is a big undertaking, so it needs sufficient budget and resources to be effective. The exercise will also undoubtedly flag some issues for remediation, which will need additional resources and investment to resolve, but that shouldn’t be a deterrent. Model risks that crystallise can have significant consequences that outweigh investment in model-risk controls and assurance processes. You also need to consider how you’ll balance baselining in the first line, with model risk assurance in the second. As insurance firms continue to build their data science capabilities, data, and model issues could become quicker to spot. Over time, this could reduce the cost of baselining and give your team greater assurance over model risk.
Frequency
Firms need to decide how often to perform model baselining. While resourcing will be one factor, it could also impact your audits and reduce the cost. Auditors often ask for results of baselining activity, so a recent baseline can be helpful – especially as most firms change auditors every few years. Frequency really depends on your model-risk appetite and your unique risk profile. You may also want to undertake ad-hoc baselining in response to major model changes, system migrations, or product launches.
Governance
Good governance is essential to manage both model risk and the business processes that surround it. As such, it’s important to regularly review your model-related business processes to make sure they remain up to date and fit for purpose. Strengthening these governance and oversight processes, will keep insurers in step with banks – and ready for when regulatory scrutiny inevitable turns to the insurance sector.
Model baselining is nothing new and has been a mainstay of risk management to make sure models are appropriate and fit-for-purpose. Investing time and resources to baselining your models will offer your stakeholders greater assurance over their use.
There are a number of other activities related to baselining that you may need to consider, including:
- assessing your current baselining approach and governance processes; drawing on external expertise where necessary to help benchmark good practice
- identifying the models you’d like to baseline, and identify the expertise and resources needed
- developing risk appetite statements and key risk indicators to monitor your model risk controls
- testing the maturity of your model risk management framework, governance, and oversight processes.
Over time it’s also important to review your baselining processes to ensure they're appropriate for current business needs. This is particularly important for legacy products, which can get left behind due to poor data and legacy systems. You should also consider your broader model inventory, using it to identify other types of models to baseline – here we’ve been looking at the best liability model, but manuals and capital models should be considered too.
For more insight and guidance, get in touch with Simon Perry, Ken Man, Klass DeVries, and Penny Street.