Our Services
Our progressive thinkers offer services to help create, protect and transform value today, so you have opportunity to thrive tomorrow.
-
Governance advisory
We guide boards and management teams in frameworks, team processes and leadership dynamics to deliver sustainable value.
-
Financial services advisory
Get market-driven expertise to achieve your goals in banking, insurance, capital markets, and investment management.
-
Business risk services
Our market-driven expertise helps firms keep growing and manage risk in an evolving regulatory landscape.
-
Risk
Meet risks with confidence and transform your business – we support you to manage risk and deliver on your goals.
-
Economic consulting
Bespoke guidance grounded in complex economic theory and practical sector insight to help you make the right decisions.
-
Government and public sector
Experience and expertise in delivering quality public sector advisory and audits.
-
Business consulting
Partnering with you to deliver sustainable business change that helps you realise your ambitions.
-
Transaction advisory services
Whether buying or selling, we help you get the deal done with our comprehensive range of transaction advisory services.
-
CFO solutions
Our CFO solutions team can support your finance function with the flexible resource they need to get results.
-
Corporate finance advisory
Building a business is never easy. We help you maximise the value of your business and find the right option.
-
Valuations
Help to understand or support the valuation of a business or asset.
-
Insolvency and global asset recovery
We provide asset tracing and seamless cross-border global recovery for clients.
-
Forensic and investigation services
Market-driven expertise in investigations, dispute resolution and digital forensics.
-
Restructuring
Our restructuring team help lenders, investors and management navigate contingency plans, restructuring and insolvency.
-
Transformation consulting
Is business transformation a priority for your organisation? Our expert insight and guidance can help you achieve it.
Experience our agile audit approach for market-leading quality, tailored expertise, and efficient digital enablement.
-
Pensions assurance
A tailored service that responds to evolving risks and regulations.
-
Accounting services
Optimise your growth with expert accounting services. Contact us today.
-
Royalty and intellectual property (IP) audits
Enhance IP asset protection with our royalty and IP audit services. Expertise in licensing, revenue detection, and compliance improvements.
Related insights:
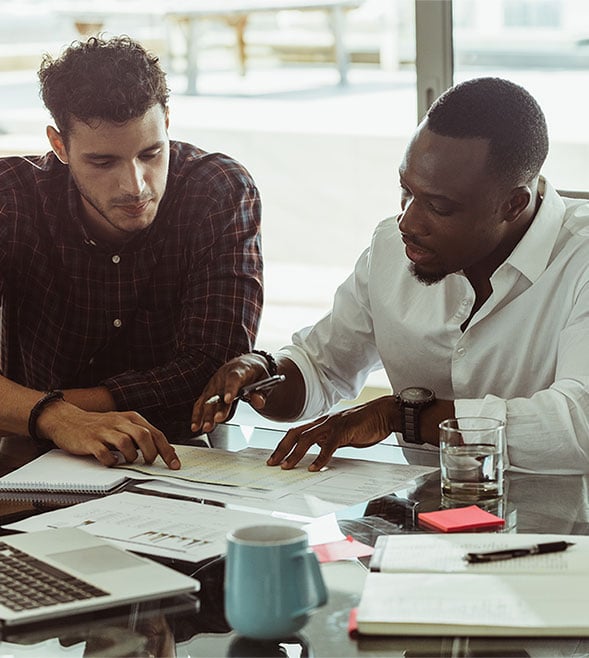
Article
What's your ECCTA ‘failure to prevent fraud’ action plan?
If you haven’t already started building your ‘reasonable procedures' defence for ‘failure to prevent fraud’ then you need to get going on it.
Seize new opportunities, and navigate through any complexities and risks
-
Business consulting
Partnering with you to deliver sustainable business change that helps you realise your ambitions.
-
CFO solutions
Our CFO solutions team can support your finance function with the flexible resource they need to get results.
-
Corporate Simplification
Release value, reduce compliance complexity, and improve tax efficiency by streamlining your group structure.
-
Economic consulting
Bespoke guidance grounded in complex economic theory and practical sector insight to help you make the right decisions.
-
Governance advisory
We guide boards and management teams in frameworks, team processes and leadership dynamics to deliver sustainable value.
-
International
Unlock global opportunities with our local expertise and worldwide reach.
-
People advisory
Driving business performance through people strategy and culture.
-
Strategy Group
Successful business strategy is rooted in a clear understanding of the market, customer segmentation and how purchase decisions vary.
Cyber crime is on the rise and you need to protect your business. We offer tailored security solutions for your firm.
-
Respond: Data breach, incident response and computer forensics
Are you prepared for a cyber failure? We can help you avoid data breaches and offer support if the worst happens.
-
Comply: Cyber security regulation and compliance
Cyber security regulation and compliance is constantly evolving. Our team can support you through the digital landscape.
-
Protect: Cyber security strategy, testing and risk assessment
Cyber security threats are constantly evolving. We’ll work with you to develop and test robust people, process and technology defences to protect your data and information assets.
Related insights:
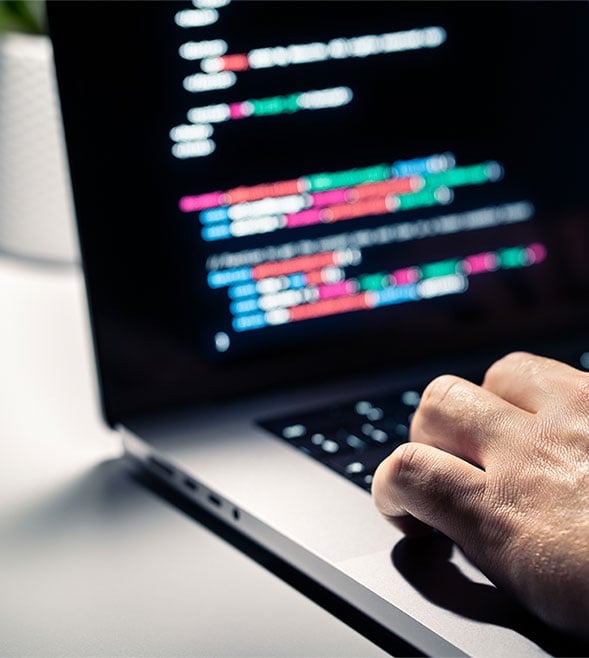
Article
The rise of hypervisor targeting: how can retail respond to Ransomware?
Ransomware attacks are evolving. How can you strengthen your defences to ensure your business stays protected?
Supporting you to grow your business profitably through our partner-led deals service.
-
Corporate finance advisory
Building a business is never easy. We help you maximise the value of your business and find the right option.
-
Debt advisory
Working with borrowers and private equity financial sponsors on raising and refinancing debt. We can help you find the right lender and type of debt products.
-
Financial modelling services
Financial modelling that helps you wrestle with your most pressing business decisions.
-
M&A data analytics
We transform and visualise data to present meaningful and clear outputs, enabling you to make better decisions and realise greater value.
-
Operational deal services
Enabling transaction goals through due diligence, integration, separation, and other complex change.
-
Our credentials
Search our transactions to see our experience in your sector and explore the deals advisory services we've delivered.
-
Transaction advisory services
Whether buying or selling, we help you get the deal done with our comprehensive range of transaction advisory services.
-
Valuations
Help to understand or support the valuation of a business or asset.
Our pragmatic approach will help you maximise your ESG strategy and reporting.
-
The ESG agenda
Shape your ESG agenda by identifying the right metrics, sustainable development and potential business value impact.
-
ESG driven business transition
Whatever your ESG strategy, we can support your organisation as it evolves while maximising efficiency and profitability.
-
ESG programme and change management
Do you have the right capabilities to drive the delivery of your ESG strategy to realise your targets?
-
ESG risk management
You must protect, comply, understand and influence to successfully manage the risk involved with ESG issues. We can help.
-
ESG strategy, risk and opportunity identification
We can help you clearly define your ESG Strategy, with the risks and opportunities identified and managed.
-
Create value through effective ESG communication
Building trust and engagement with your stakeholders on your ESG strategy.
-
ESG metrics, targets and disclosures
The pressure to report your ESG progress is growing. Do your targets measure up?
-
ESG governance, leadership and culture framework
Make the most of ESG opportunities by effectively embedding your strategy across your organisation.
-
ESG and non-financial assurance
Support your board to be confident in supplying robust information that withstands scrutiny.
-
Transition planning to net zero
Supporting your organisation in the transition to net zero.
Get market-driven expertise to achieve your goals in banking, insurance, capital markets, and investment management.
-
Actuarial and insurance consulting
We consult extensively to the life insurance, general insurance, health insurance and pensions sectors.
-
Business risk services
Our market-driven expertise helps firms keep growing and manage risk in an evolving regulatory landscape.
-
Financial crime
Helping you fight financial crime in a constantly changing environment
-
Financial services business consulting
Leverage our diverse capabilities to manage challenges and take opportunities: from assurance to transformation
-
Financial services tax
Helping financial services firms navigate the global financial services and funds tax landscape.
-
Regulatory and compliance
Providing an exceptional level of regulatory and compliance to firms across the financial services industry.
Market-driven expertise in investigations and dispute resolution.
-
Corporate intelligence
Corporate intelligence often involves cross-border complexities. Our experienced team can offer support.
-
Litigation support
Industry-wide litigation support and investigation services for lawyers and law firms.
-
Disputes advisory
Advising on quantum, accounting and financial issues in commercial disputes.
-
Forensic investigations and special situations
Do you need clarity in an uncertain situation? If you're accused of wrongdoing we can help you get the facts right.
-
Forensic data analytics
Our forensic data analytics team are helping businesses sift the truth from their data. See how we can help your firm.
-
Monitoring trustee and competition services
Monitoring trustee services to competition, financial and regulatory bodies.
-
Financial crime
Supporting your fight against financial crime in an ever-changing environment
-
Whistleblowing and investigation support
Whistleblowing frameworks provide many benefits – find out how to build trust and manage risks in a confidential, cost-effective, robust way.
Experience and expertise in delivering quality public sector advisory and audits.
-
Public sector advisory
To deliver excellent public services, local and central government need specialist support.
-
Public sector consulting
Helping public sector organisations maintain oversight of services and understand what's happening on the ground.
-
Public sector audit and assurance
As a leading UK auditor, we have unparalleled insights into the risks, challenges and opportunities that you face.
We provide asset tracing and seamless cross-border global recovery for clients.
-
Competition damages and class actions
Helping you recover damages owed from anti-competitive practices.
-
Contentious estates and family disputes
We manage complex and sensitive disputes through to resolution.
-
Digital Asset Recovery
Get guidance and technical expertise on digital finance and cryptoasset recovery from our dedicated crypto hub.
-
Grant Thornton Offshore
Grant Thornton Offshore is our one-stop global solution for insolvency, asset recovery, restructuring and forensics services.
-
Insolvency Act Portal
Case information and published reports on insolvency cases handled by Grant Thornton UK LLP.
-
Litigation support
Industry-wide litigation support and investigation services for lawyers and law firms.
-
Personal insolvency
We can support you to maximise personal insolvency recovery and seek appropriate debt relief.
Unlock global opportunities with our local expertise and worldwide reach
-
South Asia business group
We help Indian companies expand into the UK and invest globally. We also help UK companies invest and operate in India.
-
US business group
Optimise your trans-Atlantic operations with local knowledge and global reach.
-
Japan business group
Bridging the commercial and cultural divide and supporting your ambitions across Japan and the UK.
-
Africa business group
Connecting you to the right local teams in the UK, Africa, and the relevant offshore centres.
-
China-Britain business group
Supporting your operations across the China – UK economic corridor.
Our restructuring team help lenders, investors and management navigate contingency plans, restructuring and insolvency.
-
Asset based lending advisory
Helping lenders, their clients and other stakeholders navigate the complexities of ABL.
-
Contingency planning, administrations and liquidations
In times of financial difficulty, it is vital that directors explore all the options that are available to them, including having a robust ‘Plan B’.
-
Corporate restructuring
Corporate restructuring can be a difficult time. Let our team make the process simple and as stress-free as possible.
-
Creditor and lender advisory
Whether you're a creditor or lender, complex restructurings depend on pragmatic commercial advice
-
Debt advisory
Our debt advisory team can find the right lender to help you in restructuring. Find out how our experts can support you.
-
Financial services restructuring and insolvency
Financial services restructuring and insolvency is a competitive marketplace. Our team can help you navigate this space.
-
Pensions advisory services
DB pension-schemes need a balanced approach that manages risk for trustees and sponsors in an uncertain economy.
-
Restructuring and insolvency tax
Tax will often be crucial in a plan to restructure a distressed business. Our team can guide you through the process.
-
Restructuring Plans
Market leading experience in advising companies and creditors in Restructuring Plan processes.
Related insights:
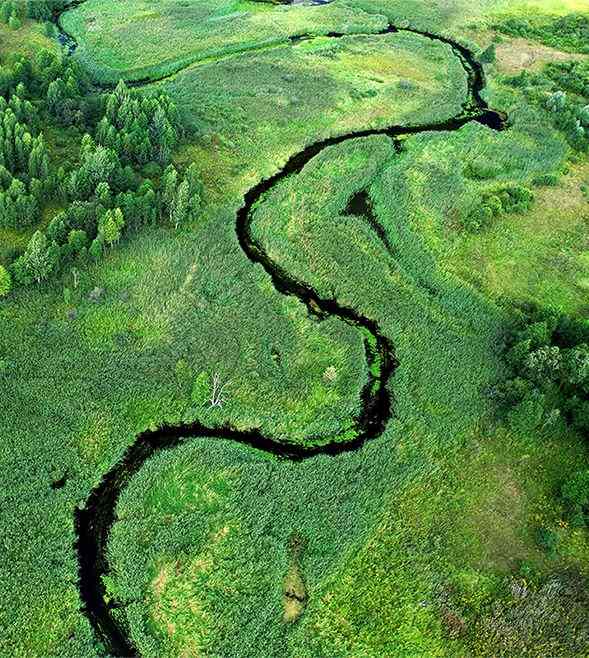
Article
PRA proposals on climate risk could raise cost of borrowing
If lenders have to integrate climate risks into their capital adequacy framework for loans, this could be a pivotal moment for borrowers.
Meet risks with confidence and transform your business – we support you to manage risk and deliver on your goals.
-
Artificial intelligence
Our approach to the design, development, and deployment of AI systems can assist with your compliance and regulation.
-
Controls advisory
Build a robust internal control environment in a changing world.
-
Data assurance and analytics
Enhancing your data processes, tools and internal capabilities to help you make decisions on managing risk and controls.
-
Enterprise risk management
Understand and embrace enterprise risk management – we help you develop and connect risk thinking to your objectives.
-
Internal audit services
Internal audit services that deliver the value and impact they should.
-
Managing risk and realising ESG opportunities
Assess and assure risk and opportunities across ESG with an expert, commercial and pragmatic approach.
-
Project, programme, and portfolio assurance
Successfully delivering projects and programmes include preparing for the wider impact on your business.
-
Service organisation controls report
Independent assurance provides confidence to your customers in relation to your services and control environment.
-
Supplier and contract assurance
Clarity around key supplier relationships: focusing on risk, cost, and operational performance.
-
Technology risk services
IT internal audits and technology risk assurance projects that help you manage your technology risks effectively.
Related insights:
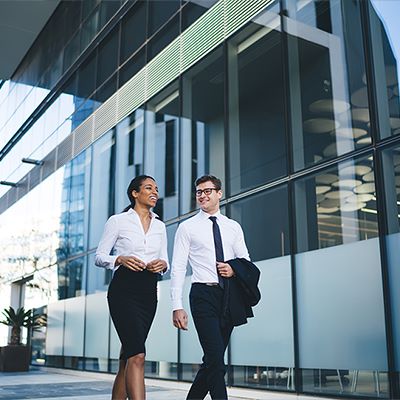
Article
FCA’s section 165 request signals strategic shift
FCA’s Section 165 request marks a shift to data-led supervision. Learn what firms must do to align with Consumer Duty and stay compliant.
Tax has always been complicated, but it's more important than ever to understand how the evolving tax landscape impacts you and your business.
-
Capital allowances (tax depreciation)
Advisory and tools to help you realise opportunities in capital allowances.
-
Corporate tax
Helping companies manage corporate tax affairs: delivering actionable guidance to take opportunities and mitigate risk.
-
Employer solutions
We will help you deliver value through your employees, offering pragmatic employer solutions to increasing costs.
-
Financial services tax
Helping financial services firms navigate the global financial services and funds tax landscape.
-
Indirect tax
Businesses face complex ever changing VAT regimes, guidance and legislation. We can help you navigate these challenges.
-
International tax
Real-world international tax advice to help you navigate a changing global tax landscape.
-
Our approach to tax
We advise clients on tax law in the UK and, where relevant, other jurisdictions.
-
Private tax
Tax experts for entrepreneurs, families and private business. For now and the long term.
-
Real estate tax
Stay ahead of real estate tax changes with holistic, tax-efficient solutions.
-
Research and development tax incentives
We can help you prepare optimised and robust research and development tax claims.
-
Tax dispute resolution
We make it simple to stay compliant and avoid HMRC tax disputes
-
Tax risk management
We work with you to develop effective tax risk management strategies.
The pace of digital change creates opportunities and risks. Our experts can help your business transform with confidence.
-
Digital assets
As frontrunners in digital asset advisory, we’re here to help you navigate both the opportunities and challenges in this unique asset class.
Helping you shape the future of your industry.
Insight and practical guidance to help upstream and downstream automotive companies adapt and grow.
Bringing insight-led guidance and diverse perspectives to help business support services achieve their ambitions
-
Skills and training
Get the right support to deliver corporate and vocational training that leads the way in an expanding market.
-
Private education
Insight and guidance for all businesses in the private education sector: from early years to higher education and edtech.
-
Facilities management and property services
Get insight and strategic support to take opportunities that protect resilience and drive UK and international growth.
-
Recruitment
Helping recruitment companies take opportunities to achieve their goals in a market where talent and skills are key.
There are opportunities in consumer markets. Our experience, insight, and rigorous approach can help you take them.
-
Food and beverage (F&B)
We can help you find the right ingredients for growth in your food and beverage business.
-
Travel, tourism and leisure
Tap into our range of support for travel, tourism and leisure businesses in this period of challenge and change.
-
Retail, e-commerce and consumer products
With multiple challenges and opportunities in the fast-evolving retail sector, make sure you are ready for them.
Adapting to an evolving market is tough. We support clients across the whole energy sector to adapt, succeed and grow.
Regulatory change, pressure on cost management and growth, and increased investment in technology and data are dominating the financial services industry.
-
Banking
Our expertise and insight can help you respond positively to long term and emerging issues in the banking sector.
-
Capital markets
2020 is a demanding year for capital markets. Working with you, we're architecting the future of the sector.
-
Insurance
Our experienced expert team brings you technical expertise and insight to guide you through insurance sector challenges.
-
Investment management
Embracing innovation and shaping business models for long-term success.
-
Pensions
Pension provision is an essential issue for employers, and the role of the trustee is becoming increasingly challenging.
-
Payments advisory and assurance
Payment service providers need to respond to rapidly evolving technical innovations and increased regulatory scrutiny.
Related insights:
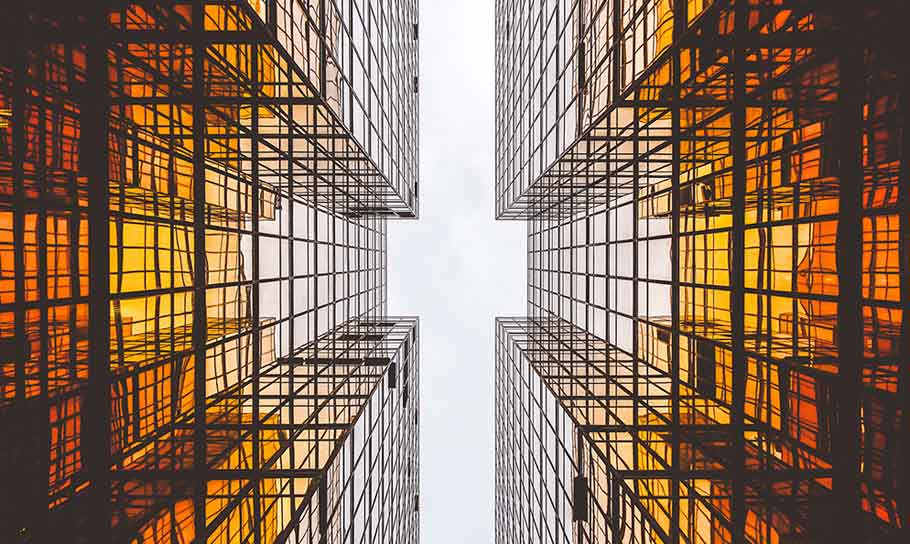
Technical
Your guide to this week in regulation
Stay up to date with our latest round up of financial regulation.
The pandemic is an unprecedented challenge for the public sector. The right strategic support is vital.
-
Central and devolved government
Helping central and devolved governments deliver change to improve our communities and grow our economies.
-
Infrastructure and transport
Delivering a successful transport or infrastructure project will require you to balance an often complex set of strategic issues.
-
Local government
Helping local government leverage technical and strategic expertise deliver their agendas and improve public services.
-
Regeneration development and housing
We provide commercial and strategic advice to assist your decision making in pursuing your objectives.
-
Health and social care
Sharing insight and knowledge to deliver transformation and improvement to health and social care services.
Supporting industrials and manufacturing businesses to grow, protect and create value.
Helping charities, education, social housing and other organisations to overcome pressures and achieve their goals.
-
Charities
Supporting you to achieve positive change in the UK charity sector.
-
Education and skills
The education sector has rarely faced more risk or more opportunity to transform. You need to plan for the future.
-
Social housing
We are committed to helping change social housing for the better, and can help you make the most of every opportunity.
Related insights:
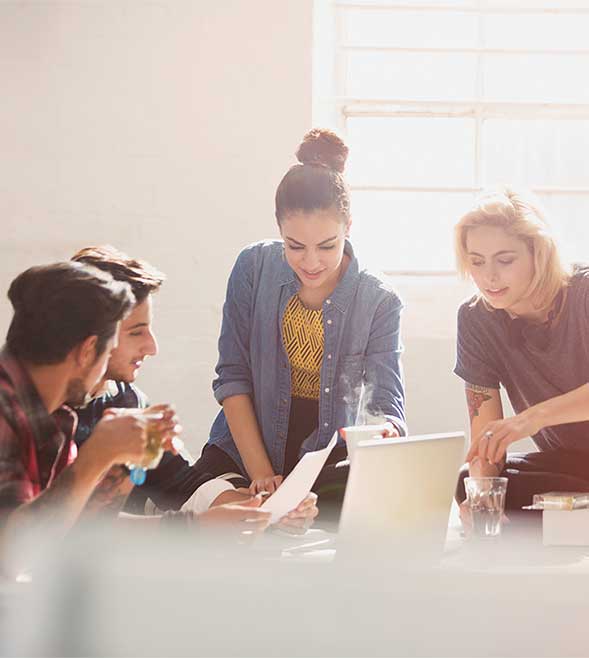
Webinar
Charity sector webinar 2025
The charity sector continues to face a difficult operating environment. Watch our latest webinar to find out how to navigate these challenges.
Our team brings clear and actionable solutions to the private sector healthcare, helping companies achieve their goals.
The expertise you need in real estate advisory, audit, and tax to get the right strategy for your business.
Are you ready to deliver on your growth ambitions? We work with TMT leaders to navigate the risks and opportunities.
-
Technology
We work with dynamic technology companies of all sizes to help them succeed and grow internationally.
-
Telecommunications
Take all opportunities to realise your goals in telecommunications: from business refresh to international expansion.
-
Media
Media companies must stay agile to thrive in today’s highly competitive market – we’re here to support your ambitions.
Discover more than you expected with a career at Grant Thornton.
Looking for a more fulfilling role in professional services? One where fresh thinking, collaboration and diversity are valued? At Grant Thornton we do things...
-
Career development
The support you need will vary throughout your career, here are just some of the ways we'll support you to you excel with us.
-
How we work
Our approach to how we work, ensures that when we are making choices about how, where or when we work, we have the support and tools we need.
-
Reward and benefits
We are committed to building a culture where our people have access to the necessary benefits to help promote a healthy lifestyle and thrive.
-
Inclusion and diversity
Included and valued for your difference is how everyone should feel at work. Not just because it’s right, but because we’re all at our best when we’re able to be ourselves.
-
ESG: environment and community impact
Our ESG framework enables responsible, sustainable, and ethical operations. We prioritise the environment, our broader societal impact, and our firm's governance to protect the planet, foster inclusivity and wellbeing, support our communities, and bolster our firm's resilience.
Everything you need to know about our apprentice and graduate early careers programmes at Grant Thornton.
-
What we do
It’s an exciting time to be joining Grant Thornton, especially as a trainee at the start of your career. Learn about our teams and the work we do.
-
Life as a trainee
Everything you need to know about life as a trainee, from the experiences you'll get to the skills you need.
-
Employability hub
Our employability hub is designed to help you feel prepared for the application process, and guide you through the decisions you will need to make throughout.
-
Our programmes
Whether you are looking to join us straight from school or with a degree, or even looking for some work experience, we have a programme that is right for you.
-
Parents, carers and teachers hub
We've created this hub to provide you with the information you'll need to help inform career discussions with your young people. From whether an apprenticeship is the right route and the application process, to the support they'll receive and future career opportunities, this page will help you guide informed decisions.
-
FAQs
We hope you can find all the information you need on our website, but to help we've collated a few of the questions we hear quite frequently when speaking to candidates.
Looking for a more fulfilling role in professional services? One where fresh thinking, collaboration and diversity are valued? At Grant Thornton we do things...
-
Advisory
Our advisory practice provides organisations with the advice and solutions they need to unlock sustainable growth and navigate complex risks and challenges.
-
Audit
Every day our audit teams help people in businesses and communities to do what is right and achieve their goals.
-
Tax
The tax landscape is evolving, and our clients need us more than ever to navigate the complexities with them. Our firm has seen remarkable growth in recent years and we have set ambitious plans for the future. To achieve them, we're dedicated to developing our internal talent and excited to welcome new team members who want to grow with us.
-
Central Client Service
From finance and information systems, to marketing and people teams we have a diverse range of teams supporting our business.
-
Our recruitment process
We strive to ensure our interview process is barrier free and sets you up for success, as well as being wholly inclusive and robust. Learn about our process here.
-
International hiring
Our international talent is an important part of our firm, joining us you will be joining a growing community of international hires.
-
Armed forces
As an Armed Forces Friendly organisation we are proud to support members of the Armed Forces Community as they develop their career outside of the Armed Forces.
Are you a partner or director, or on track to one of these positions? Are you looking for a more exciting role where you can drive change and help shape the...
The Agile Talent Community is a network of contract professionals given the opportunity to work with valued clients on a project-by-project basis and provided...
Experienced professionals job opportunities with Grant Thornton
Experienced professionals job opportunities with Grant Thornton